How to integrate remote sensing into the forest carbon market, the right way
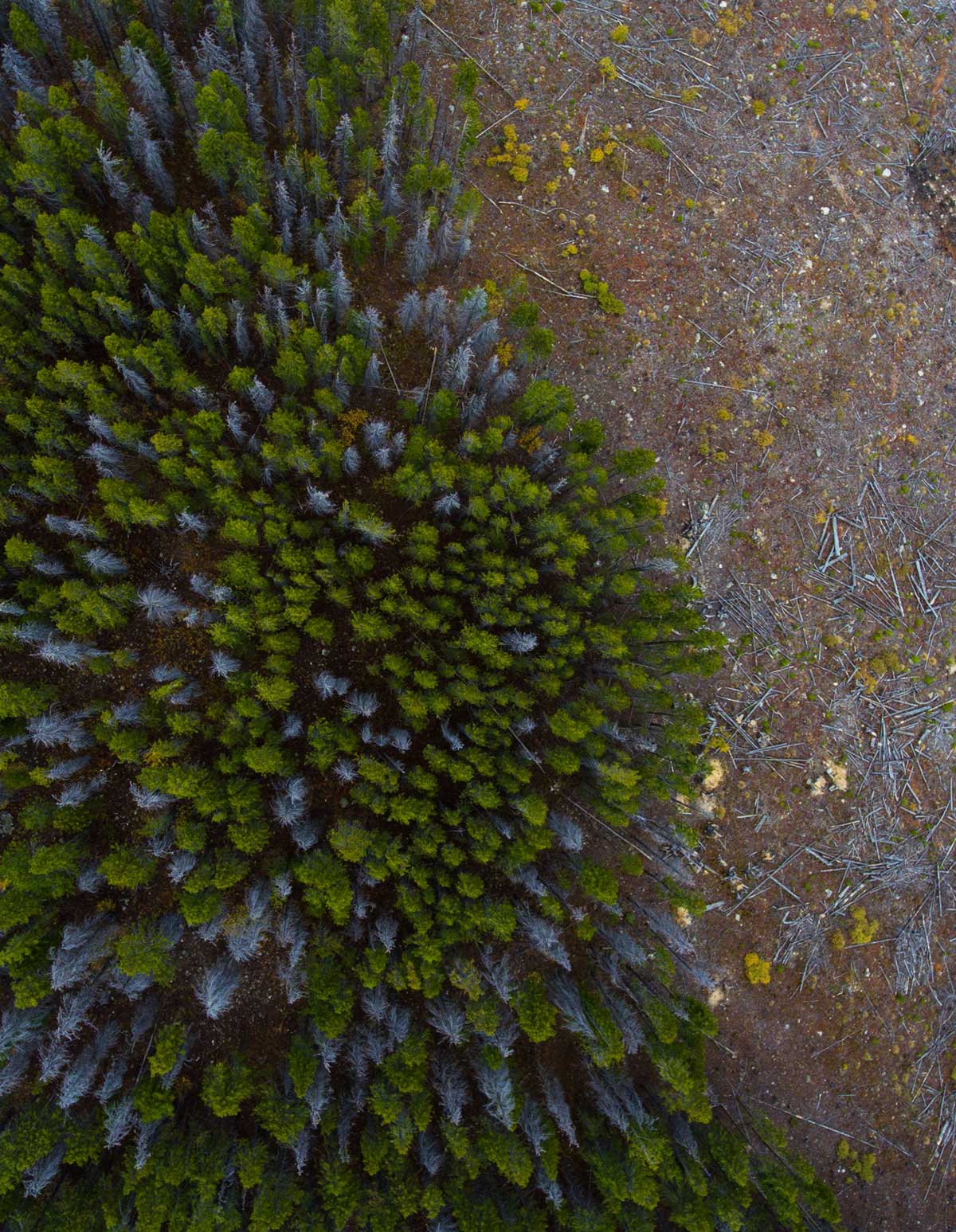
By Andrew Taylor, Senior Technical Manager, Forestry
The forest carbon market quantifies and monetizes the climate benefits of preserving and growing healthy forest ecosystems. The industry has seen rapid growth in recent years and is demanding quality and scalability. Standards bodies, such as the American Carbon Registry (ACR), the world’s oldest private greenhouse gas (GHG) registry, are gatekeepers for quality assurance and rigor in this system. Anyone familiar with the forest carbon market would agree that projects must jump through many ‘hoops’ and meet rigorous criteria to get credits issued.
Offset buyers have always demanded rigor and integrity, and the basis for determining what makes a high-quality, fully vetted forest carbon project merges several branches of science, such as forest ecology, economics, and statistics. The increasing relevance of carbon credits in a global economy weening itself off fossil fuels has put forest carbon projects under a microscope, with more and more credit rating agencies and academics now dedicated to reviewing forest carbon claims. By and large, the forest carbon market has responded by tightening its requirements ever more in an earnest effort to continuously improve, albeit too slowly for some critics.
Increased rigor and integrity, however, come at a cost. The more checkboxes there are, the more expensive each forest carbon project becomes. This has proved frustrating to many interested in quickly scaling the market to achieve the pace necessary to mitigate the worst effects of climate change. There have also been long-standing demands to increase accessibility to carbon finance for all landowners, particularly small family forestland owners who own a majority of private forestlands in the United States. While ACR has taken steps to alleviate barriers to traditionally excluded landowners (such as developing a forest carbon methodology specifically for small landowners), market access and scientific rigor can still sometimes seem at odds. Perhaps complexity and expense come with the territory of high-quality forest carbon projects. More optimistically, perhaps innovation can help deliver high-quality carbon projects with reduced barriers to entry.
A myriad of remote sensing technologies has recently emerged, offering the promise to transform the forest carbon space by taking measurements at scale for a fraction of the cost of traditional measurements. Scientists and entrepreneurs alike are realizing the potential of these technologies, such as light detection and ranging (Lidar) and high-resolution satellite imagery, often paired with machine learning algorithms, to measure forests and their climate benefits. Many new companies are looking to deploy remote sensing to develop carbon offset projects.
Figure: An artist’s conception of the Landsat 9 spacecraft, a satellite-mounted passive sensor collecting optical data and producing medium-resolution imagery, high above the US Gulf Coast. Image courtesy of USGS/NASA Landsat.
Historically, forest carbon projects have relied on foresters to visit field plots and measure tree heights and diameters, which are used to calculate carbon. While foresters can’t feasibly measure every tree in the forest, there are tried and true statistical approaches that allow us to estimate a land’s total carbon from a set of plots with a known degree of certainty. The more area measured and the less variable the measurements, the more certain we are of the final carbon estimate. With this approach, uncertainty can be quantified and used to reduce the number of credits earned by a project, if it doesn’t meet a certain threshold. In fact, quantifying and deducting for uncertainty has become a basic tenet of forest carbon projects, preventing over-crediting and providing carbon offset buyers assurance that their credits are having the climate impact they claim.
Another basic tenet of the carbon market is verification by a reliable third-party expert. For decades traditional forest carbon verifications have involved visiting a subset of field plots and checking their independent measurements against the forester’s original measurements. The project’s inventory is only accepted if it is statistically equivalent to the verifier’s remeasurements. In addition to replicating field measurements, verifiers check each and every aspect of a project that affects the legitimacy and accuracy of their final carbon claim. This system provides carbon offset buyers yet another layer of quality assurance.
If the carbon market has come to expect third-party verification and uncertainty deduction as guarantors of carbon credit quality, then logically we must find a way to apply them to remotely sensed forest carbon projects too. But, there are serious complexities with verifying remotely sensed forest inventories and quantifying their uncertainty. In fact, a standardized approach is yet to emerge.
The most common means of leveraging lidar and satellite measurements in the carbon market involves a process of modeling, often calibrated by pairing imagery or remotely sensed estimates with a limited set of field plots. Techniques from each company are unique and are more often than not proprietary. Carbon from the same forest can be calculated ten different ways. Who is to say which is the “correct” answer?
We can’t expect verifiers to have the technical knowledge, or the time needed to understand and proof each company’s proprietary model, let alone troubleshoot it. Following the calculations from end to end means not only understanding the satellite or measurement technologies, but more dauntingly, understanding the computer programming that compiles raw measurement data into project-wide carbon estimates. To verify the process such that raw data can be traced to final carbon calculations would be essentially learning a new software product for each company. This firmly falls outside of the wheelhouse and duties of the already busy forest carbon verification industry. Even if verifiers could fully grasp a company’s technique, a standardized way of assessing its appropriateness and legitimacy is yet to emerge.
It also seems inappropriate for standards bodies to give single companies their universal stamp of approval. Just like the verifiers, the standards bodies cannot possibly take the time to vet each proprietary technique (and further have no agreed upon criteria to base such an assessment). Approving individual companies would give unfair market access at the expense of their competitors, effectively picking winners and losers.
By its very nature, a proprietary technique leaves some component of the carbon quantification undisclosed. This means offset buyers and watchdogs are left in the dark on specifics of how the final carbon estimates are calculated. That’s no way to facilitate an open market. What’s needed is a framework for objectively assessing remotely sensed carbon estimates against an accepted source of truth that ideally could be universally applied and compared regardless of the technological approach.
ACR is developing just such a framework right now. We envision two pathways for verifying remotely sensed carbon estimates: through field measurements, or through a publicly available “benchmarking” platform. First, let’s discuss field measurements.
ACR is currently working to establish specifications for field data collection within a carbon project specifically tailored to assessing the accuracy of remotely sensed carbon estimates. Our framework will specify the statistical tests and thresholds for accepting or rejecting remotely sensed carbon estimates. We envision this would involve evaluating the actual output of each remotely sensed model, rather than simply assessing model fit with more commonly used “leave-one-out” model validation approaches. After all, we’re concerned with the “final answer” that determines crediting, not just the interim steps involved to develop and train each model. The idea is that if field measurements within a carbon project are reasonably aligned with a project’s remotely sensed output, then we can reach sufficient assurance that the remote sensing technique for that project is valid.
Next, let’s discuss the concept of a publicly available benchmark. Unlike proprietary techniques that are often a “black box”, what if a public entity, such as a US federal agency, maintained a fully transparent, scientifically vetted platform for remotely estimating carbon across the country? This could provide another accepted source of truth, or benchmark, that could be used to estimate carbon within each project. The benchmark could theoretically be used on its own, or as a comparison tool to evaluate the efficacy of other proprietary approaches. Just like for field measurements, we envision a framework that would set statistical criteria for accepting or rejecting the project’s remotely sensed carbon estimates. While such a benchmarking platform doesn’t exist yet, ACR is engaging with stakeholders and researchers to help make it a reality.
Through this framework, we’ll offer equal footing to all market participants who wish to utilize remote sensing in forest carbon projects. Verifiers won’t be expected to learn the ins and outs of each proprietary technique, and instead they can continue doing what they do best: objectively assessing forest carbon inventories with statistical tests and evaluating critical components of carbon projects first-hand. Lastly, and perhaps most importantly, this will allow for scaling the market while still offering offset buyers the assurance of knowing that each carbon claim adheres to a commonly accepted threshold of accuracy.
And, what about deducting for uncertainty? While the uncertainty of “traditional” field sampling of forest inventory plots is statistically proven, the uncertainty associated with remotely sensed carbon estimates is fundamentally different. In fact, we’ve observed that there is not yet clear consensus on estimating “uncertainty” in the remote sensing industry and approaches often vary from technique to technique. Until clear scientific consensus emerges, we plan to explore indisputably conservative approaches to mitigate the uncertainty of remotely sensed carbon estimates. Several carbon methodologies have used such conservative measures to provide confidence in their final carbon estimates when uncertainty quantification just isn’t possible. Determining exactly how to treat the uncertainty of remotely sensed estimates is a major focus of our framework development process.
Figure: Comparison (left to right) of Landsat 8 at 30 m, WorldView2 at 0.5 m, and drone imagery at 0.07 m demonstrating how data resolution, quality, and geographic scale can affect the outcome of remotely sensed carbon estimates. Image from Remote Sensing in Ecology and Conservation Blog (rsecjournal.blog).
As a standards body, ACR could take the easy path of one-off approval of remote sensing companies for carbon project development. This is tempting, because it’s truly stunning what emerging technology is capable of, which is becoming less expensive and more commercially available every year. There really are existing ways to estimate forest carbon from space and from planes, and they’re ready to be deployed. But tech solutions in the carbon space deserve the same level of rigor and transparency expected in other areas of the market. ACR is committed to opening the door to remote sensing technologies, while creating a level playing field for all actors, maintaining market integrity, and providing the quality assurance that ACR has worked towards for decades.
If you share our vision and would like to contribute your expertise in remote sensing, forest carbon, or statistics, please reach out. ACR is seeking partners to provide input as we develop our remote sensing framework.